What's going on with AI in the world today?
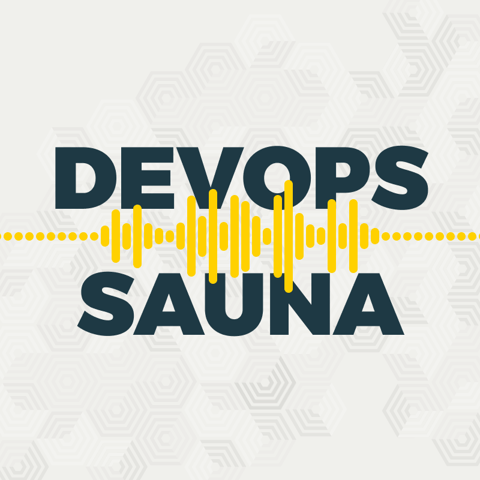
Since early 2023, everyone has been scrambling around AI, although there have been discussions around it even before ChatGPT and GPT models came out. What's going on with AI in the world today? Is this a hype cycle? Is this something real? Jaakko Vainio, COO of Silo AI, is here to discuss this with us, as he's been in the game for a little while.
Jaakko (00:07): You cannot really know in advance what you're wanting, so you have to say it. In that sense, it's not so different to humans. You have to communicate with it.
Marc (00:21): This season, Andy and Marc are back with a fantastic group of guests.
Andy (00:26): I bet the depths remain classified. And Marc keeps his head in the clouds. With our combined experience in the industry, we can go from the bare metal to the boardroom. Enjoy your time in the DevOps Sauna.
Marc (00:46): Hello, we are back in the sauna. I am super excited to keep talking about what's going on with AI today. I've got my usual cohort Andy Allred.
Andy (00:56): Hello-hello. Happy to be here.
Marc (00:56): Awesome. And we're super excited to have a guru in the house today, Jaakko Vainio, the COO of Silo AI. Hello, Jaakko.
Jaakko (01:08): Hello. Thanks for the invitation.
Marc (01:10): Super cool to have you here. I've been following the work that Silo AI does for a long time. I have some friends over there. And it's super nice to have you today. Because what is going on with AI? Everybody is talking. Is this a hype cycle? Is this something real? But Jaakko, can you tell us? You've been in the game for a little while. What's going on today with AI in the world?
Jaakko (01:31): Sure. Well, there's actually quite a lot going on. And of course, everyone is talking about AI. And everyone was talking about AI even before ChatGPT and GPT models came out. But it added a new layer to the way of things getting on. And basically, five years ago, there was a lot of hype around AI, everyone was talking about doing AI, but actually, not everyone was doing AI. It was talk but not so much walking. And I would say that that's one of the things that changed. Right now, and since around March, everyone has been scrambling around to get actually doing also things related to AI. That's one of the momentum things that's been going on. And, of course, the key thing that caught the attention of everyone was related to ChatGPT. It's maybe not the most advanced model, actually, then came GPT 4 and other things. Things have really picked up. And I would say that two previous couple of months to get new, exciting technological papers and a result is now happening more than once a week. The pace has really picked up. But what I think that is one of the key reasons why ChatGPT was so successful and caught the attention of everyone was that it was in a way, the first time that for the wider audience, AI lived up to the expectations that people were having, basically coming from science fiction. It's something that you can talk to and it responds in a meaningful way. And you can have a chat on different topics. Actually, the context that it might be saying are not always that relevant, and things like that, but it seems to be something new, something that actually works. And it can be helpful in so many different places. That's what changed the minds of so many people that this actually something big. And if you are not going to be using this, someone else and everyone else will be. Actually, we need to do something right now because it's available out there, you can tap into it really easily. It's going to be a game changer.
Andy (03:57): If we step back for just a second, you were mentioning that earlier there was a really exciting technology breakthrough, new white paper something every couple of months. And now it's like, at least once a week or sometimes more. Has there been some real technology changes, some kind of breakthroughs that advances that? Or is it just that it's much more exciting, so more people are doing it?
Jaakko (04:22): That's a very good question. I would say that much of it is about the attention to it. Many more people are working on it. And in a way what's also related to the success of ChatGPT and what followed is that it's the technology behind it is rather new, but it's not something spectacular compared to say GPT 3, so it's based on transformers, on attention models, and that came out a couple of years ago, so relatively new, but in the AI field, which is very fast going, it's not something brand new. But what's happened there is, I would say that ChatGPT was the user experience was great. It's easy to use. You don't have to use an API to tap into it. I mean, you can do that as well. But basically, anyone can try it out and it works. The engineering that happened on top of it is actually one of the major changes because before it was something used by researchers and people who were deep into the field, but this is something that's for everyone. And that's a very important thing. And also, that engineering happening on top of the research is part of what's giving us these new ways to use LLM, and also new LLM models. And when I say LLM, that's a Large Language Model. That's a term that's rather new. I would say that very few people, even last year, were talking about LLMs. And now it's popping up in even mainstream media. That's an example of how things are moving fast. But at the core of what an LLM or Large Language Model is. We have had language models for a long, long time. What exactly makes a large language model? What is the first L there? That's a good question, but I would say that most of these irrelevant models that are transformer-based, which is the technology, the neural architecture behind these models, most will be calling all of those nowadays, Large Language Models.
Andy (06:43): I saw an interview or I was watching the Apple Keynote, and it was interesting that they did not bring up AI. They mentioned transformers and a couple of things, but they didn't come out with the chat with AI kind of stuff that some people expected them to. But then in Good Morning America, they interviewed Tim Cook, and they were talking about so tell me about this Large Language Model. It's mainstream enough to be in Good Morning America. I was curious about your answer to that, which echoes what I was thinking. And I'm definitely not in the industry as deep as you are, but I don't see that there's been any huge changes other than ChatGPT got popular because it is pretty good. And because of that, everybody's like, "Hey, this is popular. I need to be into it. " Which means people are investing more. And since people are investing more, and people are looking for those keywords, then they're finding more. And it's just coming up. But it really is interesting how Good Morning America is talking about Large Language Models like come on, really?
Jaakko (07:51): Yeah. And continuing on the topic of what has changed. There is one side, which is like technology wise, maybe not something hugely new, again, is that what is the difference between the previous GPT model of GPT 3, which is also made by OpenAI, which then later on created ChatGPT and GPT 4 is that well, what a GPT model is. It's a transformer model and that's where the T comes from, but basically, ultimately what it does, it gets us what the next word should be. That's what it does. And it's very good at that. And it's the pricing how much is one can get out of that. And we can talk more about that later. But in a way, it's a nonsense generate. It creates very fluent, but mostly nonsense. And you cannot trust what it says. But what you do on top of that. That's where the reinforcement learning part comes in. Reinforcement learning is a term from machine learning at it's been around for a long time, but what's happened here is a technique called reinforcement learning with human feedback. Effectively, it was tested and if you try to generate some output and then which one of the different outputs is better, that is done by humans. And with that additional schooling, we get really, really good results. The analysis generator was schooled into being a tool you can chat with. That is what was done on top of that. And I think from the researcher perspective, how effective that was. That was the thing that was surprising for many, me included. That how well that actually worked because previously, that pure GPT model, the nonsense generator is very impressive, but is it very useful? Well, it can be used for drafting, but I would have been very hesitant to put one of those in, for example, chatbot, a client facing one. But when you do the reinforcement learning part on top of that, and then you actually start getting really good results.
Marc (10:21): I think there's an interesting and really important point here, which is that the more you use ChatGPT today, the more it's going to learn from you because you're reinforcing the things that you think are important that you think are correct. And so, there is this cultural thing that's going on here as well, where some of the smartest guys that I know, they come in, and they say, "Well, it's nonsense. An awful lot of the time, it looks good, but it's really confident, but it's not giving me what I look for." And they shy away. And I'm like, "No, you smart people are the ones that need to be in there and reinforcing the correct things from the machine." Can you open that up a little bit?
Jaakko (11:05): I'll maybe first take a step back and say that, generally, that's how AI and machine learning work in general. Typically, when you get the first models out, they are maybe not that useful. How one should be using AI in general. One needs to have a feedback loop. And whether it's as part of your recommendation system in your online store or system that you chat with, and want to be informative and not lying, or swearing, or anything like that. It's about providing that feedback. And in a way, if you set things up right, you have your AI system running perhaps as part of your digital product. You are gaining the advantage all the time that someone is using it or in a way you're giving it more and more data. Those organizations that get going as early as possible, do get an advantage. A part of the usage of AI is indeed about the fact that we should be giving them more of the correct answers, for sure. But also, depending a bit on where AI is used, there can be also things from the design side that one needs to take into account, maybe taking example from recommender systems. For example, when you're using Netflix, is that it's different to what people say that they are interested in. And then what they tap as things that they will be watching, and what they actually end up watching. The action and thinking can be different. And that's also one thing to take into account when creating AI solutions is that what is actually the thing that you should be following.
Marc (13:01): Okay. If we talk to the media and human that approaches Chat GPT, for example, and try to give them some tips of how to get the most out of the situation, how could we give somebody an approach. And then of course, I want to extend this up to my brilliant colleagues that are using it, but they're also sometimes getting a bit discouraged over the quality of the output. And it takes quite a bit of work to get what they're looking for. Are there some best practices, for example, for people to take it into use?
Jaakko (13:35): Yeah, that's also an interesting question. We have this new tool, and it's going to be affecting how we work in many different kinds of jobs. And it might be that there's less working some, but actually, this area is something that's brand new. For example, that there's a new job title called prompt engineer, so people who try to get the best out of using GPT type of technologies. And that's certainly something that didn't exist even like six months before. It's very interesting. But one of the key things is that one should not forget is that one can basically chat with ChatGPT. Even if you get something lousing in the first round, giving it more context into how it should would be acting. That's what can be very helpful in getting a more meaningful feedback or more meaningful results. And giving it feedback that at okay, this was nice, but it could you do it from a slightly another perspective that I'm especially interested in this and that. And there's a lot going on there. It could be for example, that if you want to draft an article, for example, it makes sense that you say that which kind of audience you are targeting, which kind of voice do you want to have, from which perspective, what kind of speaker you want to be. And that can be really helpful in setting the tone right. There's actually quite a lot of the things, but the most important thing is that you cannot really know in advance what you're wanting, so you have to say it. In that sense, it's not so different to humans. You have to communicate with it.
Andy (15:32): I was just going to say that's a whole lot like talking with humans. If I say, Jaakko, what's a movie I would like? You have no idea what kind of movies I have liked or anything. I have to give you some context that I prefer this type or that type, or I like this, I didn't like that, whatever. And then you get a little bit context, figuring out what I like, and then you're able to say, hey, based on that, I recommend this. That I work with Mark so much that I ever say, "Hey, Mark, I'm going to submit a talk for a conference, what should I talk about?" He already knows me well enough. He knows what kinds of topics he could suggest, but that's because we have this inbuilt context. And when we're talking with or chatting with ChatGPT, it feels like a human interaction. That's why people say, thank you, or I'm sorry. And all those kinds of things. And, and that's good. But then we have to remember that this thing we're chatting with doesn't know anything about us. How can we give it the maximum context possible? I'm talking to this type of audience. And this is the area I want to chat about. I want this to be formal, I want it to be very technical. And please give me a record. And then it starts to get enough of these context keys that it understands, or it has a better way to predict what the LLM should guess as the next word. And it starts to be less nonsense because of the context. And then it makes sense. And I say, this is actually really good. But it's the same thing we do with humans. I'm a little bit mixed on my feelings about this whole prompt engineering new type of role because it's not a new thing. It's what we do with people all the time. We just need to learn how to apply it. We just need to learn how to apply it here. And yes, somebody needs to do it, and somebody needs to focus on it and share best practices and stuff. But it's not anything new, it's just a new way to apply what we already do.
Jaakko (17:34): The interaction with ChatGPT. it feels human like and that's part of the part of the alali, but still, it's not a human and it works in different way. Of course, there's the part that some type of discussion, some type of behavior has been banned from it. It shouldn't be doing things like that. And the biography, the prompt engineering is getting the best out of that. It's a new technology, so people want to play it. People want to hack it and try to make it say things that it's not allowed to say, like how to make [inaudible] or something like that. And then there's an even a game where one tries to make ChatGPT reveal a password that it's been given. And it gets more and more difficult on how to get the password out. But in a way, I agree that it's about giving context, which is also something that we as humans always require. One can know it from before or if working with people you don't know that well, you require quite a bit of it. And in a way that leads to another interesting finding is that people typically are quite polite with ChatGPT. And it's also polite to you and in some situations, people have been saying that when they've been using Chat GPT as sort of a co-worker, they like it very much because it's so polite, and always answers and tries to help. It can be even better than humans in being a human.
Andy (19:19): I think that explains why Marc is using Copilot and what instead of pairing with me lately.
Marc (19:28): That's true. Are you in Scandinavia this autumn? Well, if you're not you ought to be because the world's greatest DevOps conference is coming to Stockholm in Copenhagen. I'll leave a link for you in the show notes. Now, back to the show.
Marc (19:52): One of the things I'm curious about when I'm using Copilot from GitHub is when I tell it, "Thank you," does that provide positive reinforcement?
Jaakko (20:02): Like generally, I think it won't be affecting the model that much, but within the discussion quality, it depends on the entire chat you're having with it. But if you're asking like, can you, for example, optimize this piece of code and then does something and then say, "No, you actually need it to focus on this part," then it works out on that. And then you say, "Thanks, this works," then yeah, but it depends on the entire conversation.
Marc (20:34): Let's try something with context building. Can we step wise go through how context is built within a chat? I ask a question, say, what is a good action movie or something like that? What is the context that it has for that initial question?
Jaakko (20:55): Yes. Basically, what ChatGPT especially knows. It has read most of the Internet by a certain date in 2021. There's stuff on, for example, IMDB that it is familiar with. There's a general knowledge of what movies have been out there, what has been popular in a wider audience. But for example, what your age, and occupation and things that might have an effect, that is something that it doesn't know. Basically, there's a general knowledge that's out there in the Internet is something that it would have.
Marc (21:40): Okay. What's an interesting next question, I say, "No, I don't want action movies with car chases or something." Okay. There's a very simple example. It can look at all of the data that's available freely on the internet and try to figure out if ones have car chases or not. And then word by word, it's going to build a conversation with me where it tells me, okay, here are some movies that maybe don't have car chases in them. But how can we make an example like this, where we help people understand how we build the context?
Jaakko (22:14): That's a good question. And of course, it depends quite a bit on the setup for that task at hand. But if you think about movies, you can try to give it more context from the content. It's likely that it knows about the synopsis of movies. One could go to the approach that if you like certain directors, for example, that is certainly the date of the race there or certain actors, or certain era, or even a style if there's like a sub-genre, or something like that you like quite a bit. But I would say it can go a bit deeper. But it can also go more often wrong there is that if you give what's your mood and that type of thing that I would now like to watch something that's kind of stuff a lot of explosions and things like that, or that I'm feeling down and I want to see -- that kind of stuff. It actually works better than expected, I would say. There's a lot of stuff written about different movies and the feelings they make. It has seen that kind of material. It can make a pretty decent leads and guess from that. And I think on that type of thing, there's been quite a bit of improvement on what GPT 4 can do compared to the previous ones. It's a larger model and it has seen slightly more data, or slightly is a comparative term here. But the results in any way seem to be a lot better. Yeah, it's not just something very simple anymore. It can go a bit in the opposite direction, but it's not suddenly 100% accurate.
Marc (24:11): Okay, so I could probably do something along the lines of I open a chat, and I asked for an action movie. And then I say, I don't like car chases, but I like this specific director. And I'm looking for a happy ending because I'm in a sad mood. And then I might go watch the movie, and then I could come back and reopen that chat, specifically and say, I was sad, and I watched this movie, and it made me happy. Please write a review.
Jaakko (24:42): Yes. I would expect that it would be something that you could use as a draft. And I think they're fewer and fewer people are saying that anymore. But I think that leads to the side of what's the level of creativity that one can expect out of these tools. I think that that's a very interesting topic as well. But it's very wide topic, of course. But until like, rather recently, last year or so, many people were saying that, okay, AI is going to be changing many different jobs and maybe taking out some job tasks. But what creative people do that's going to be safe. And I think many people are now questioning whether that's true because certainly there's some type of creativity there. I don't expect the next Nobel Literature Prize winners to be made by ChatGPT. One can try to ask it to create some short stories and they are not really that good, but they are in a way better than what the school kid might write, most school kids. I would say that creativity is starting to be there. How actually, original is that? That's a good question. But it's also a good question what you might create is. That's more on a philosophical level. But that's actually one of the clear use cases so far, the use the generative models is, for example, generating marketing content and drafts at the very least, and that's quite widely used already. It's actually take taking impact on the creative side.
Marc (26:30): Now, there's some things here that I've been grappling with for a while. I'm an artist. I've been around a lot of artists, and one of the things that we talk about a lot is that your style is a reflection of everything that you've ever seen and been interested in, plus something that's you and you alone. And that's an interesting combination because the you and you alone, is the missing component here whether you're doing music, or visual arts or poetry or whatever. Although the poetry that I get out of GPT 4 can be pretty astonishing, sometimes, especially if you give it best practices for writing poetry, but let's get into business a little bit. I've talked to some enterprises that have their initial policy was just to outlaw the GPT and things like this. And then the employees are doing it on their personal machines or on their phones or something like that, even within the work context. But can you at a really high level, how much leakage is there from people's prompt work into these models? And how concerned do people really need to be?
Jaakko (27:44): That's a very, very good question. And I would just say that there's no simple answer. There's a lot of stuff that one shouldn't be too concerned about. And it's also related to the fact that how actually original what you're doing really is. For example, creating rather simple web pages using something LLM based tooling for that, I would say it's totally okay. But if you're thinking about personal information, then you shouldn't be fitting that for sure. And if you're thinking about what's happening at the very core of your business, what is in a way your unfair advantage, so that you need to be careful about, and it's not probably likely that OpenAI, they do get the data, and Microsoft is closely there. It's quite unlikely that Microsoft or OpenAI would be taking your business with the data that they get, but what is a reasonable risk, and one that you need to be cautious about is that what you feed in there can be used to further improve the model and all the APIs also open not just to you, but all of your competition, so that they are getting access to what you're in that sense teaching it and that's the broad that one is to be all the companies should be taking into thought and deciding how they want to approach it. For example, there was the case of Samsung putting in stuff that led to their core business instead of a trade secret stuff, and that something that you don't want to be doing. But then again, if it's something related to a more or less open stuff that you are sharing anyway from your company or something that doesn't really give an advantage to anyone else. You are giving up a lot of efficiency if you're not using the best possible tools, so in that sense, making decisions that you are absolutely burning all the tooling, you're hurting your business. And as he said, it's very likely that people then find ways to still use them and roll it. That's typical that when you set up, for example, too tight cyber security, then people try to find ways to bypass it. And then they do actually something that that's really can be more dangerous and leakier in that sense. It's about finding that balance and being mindful of what's at the core of your business. And continuing on that a bit. For some companies, typically larger ones, it might make sense to have their own LLM built, but it's not something that you can do on your laptop. It requires quite a bit of resources, but if it's at the very core of what you do, what's your edge on the market, then it actually might be a very good idea. I would either say that why should be using both these technologies and AI in general in the context of your digital product, your retail service. Where are you actually want the advantage to be. Like I mentioned previously, when you have it up and running. And that's actually not like a super easy task. It takes many bits and pieces to get value out of AI on a scale. But when you have it up and running, you get more and more of the feedback, you understand your clients or whatever your business is better and better, it improves. You're in a positive flywheel. And that's what you want to be doing at the core of your business and not giving it to anyone else.
Marc (32:02): Excellent. Thank you so much Jaakko for joining us today. I think that was a really good summary. I've got two questions that we ask everyone that comes on the podcast. The first one I'd like to ask is Yaakko, when is the last time you tried something new and what was it?
Jaakko (32:23): That was actually related to renovation. I am doing a huge renovation at home. It was trying out new tooling for sanding the floors. Actually, it didn't go too well. I got to burn the fuses and do some stuff there. But yeah, there's a lot of stuff going on. And I would say it's at the very core of Silo values. Also, to the keep learning and I think it's applies to myself as well. That's almost every day I try to learn some new stuff, sometimes getting hurt in the process, but that's how we live and learn.
Marc (33:06): Really cool. I have to ask because I just moved into 100-year-old house, is it an old house or is it something modern?
Jaakko (33:12): Yeah, 120. Yes.
Marc (33:15): Awesome. We'll have to compare notes.
Jaakko (33:19): Yeah, indeed.
Marc (33:20): All right. Yes. And Andy, please.
Andy (33:21): Our other question is, when's the last time something really excited you? And what was it?
Jaakko (33:27): There's actually been a lot of exciting stuff happening, both at work and otherwise, but maybe picking something from this context that we have talked about related to the LLMs and GPT models. It's a scientific paper, but still something that I think even without scientific background, one can read. It's a rather long one from a team of researchers on Sparks of Artificial General Intelligence and the stuff that quite surprisingly, the models can do it. It is rather mind-blowing and exciting, so I can recommend that.
Marc (34:07): Excellent. We'll leave a link for you in the show notes. All right. Thank you once again, Yaakko. This has been a privilege to have you here and to share all of your knowledge with us today.
Jaakko (34:16): Yeah. Thank you so much for the invitation.
Andy (34:19): Thanks a lot. It's been fun.
Marc (34:21): Excellent. Okay, that's it for the sauna today. See you next time.
Andy (34:25): Bye-bye.
Marc (34:30): Before we go, let's give our guests an opportunity to introduce themselves and tell you a little bit about who we are.
Jaakko (34:36): Hello, everyone. My name is Jaakko Vainio. I'm Chief Operating Officer at Silo AI. I have background in theoretical physics and have worked on actually quite many things at the university. We founded a company to work on mobile games, but that was not the end of the story. We started working on AI and machine learning as well and a joint Silo AI as one of the first employees to work on natural language processing and recommender systems. And they also found quite a few different things to do, including finding our London office and slowly moving to another role that it's been really, really exciting to be part of a journey on growing. Also, the company and seeing the multiple ways of AI that helping our clients create value out of that.
Marc (35:26): My name is Marc Dillon. I'm a Lead Consultant in the transformation business at Eficode.
Andy (35:31): My name is Andy Allred. And I'm doing Platform Engineering at Eficode.
Marc (35:35): Thank you for listening. If you enjoyed what you heard, please like and subscribe. It means the world to us. Also check out our other interesting talks and tune in for our next episode. Take care of yourself and remember what really matters is everything we do with machines is to help humans
Published: